Predictive Analytics Capabilities
The Energy.ML platform helps operators increase production and reduce cost by quickly and accurately identifying production issues and predicting and preventing failures. Both mechanical and electrical components are monitored. Some examples of Energy.ML failure prediction and anomaly detection are shown below.
Pitch Bearing Failure Prediction
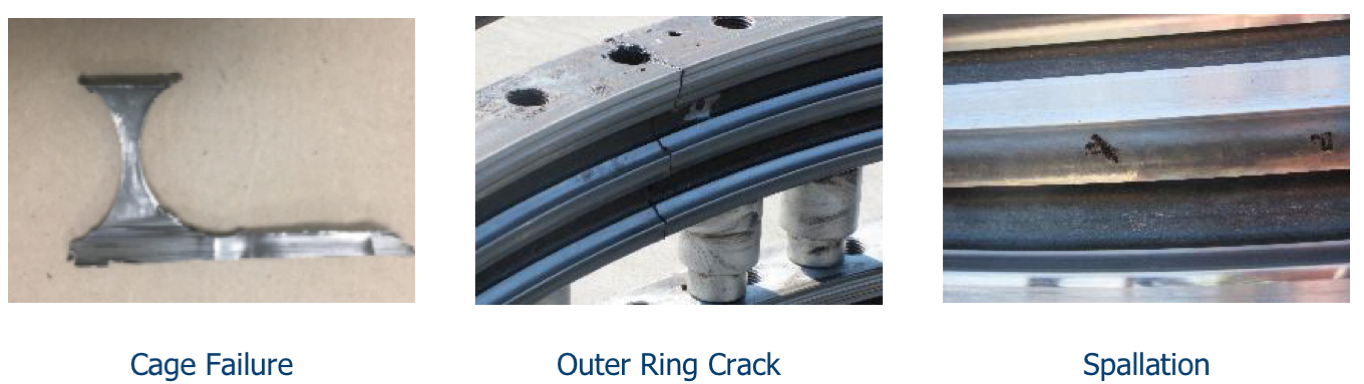
Pitch bearing failures have become increasingly common as wind turbine rotors have increased in diameter. Failure modes include cage failure, outer ring cracks, and spallation of the ball paths. These failures are costly and result in extensive machine downtime. Energy.ML can predict pitch bearing failures with high accuracy many months in advance of failure, resulting in logistics cost savings and reduced downtime.
Performance Anomaly Detection and Correction
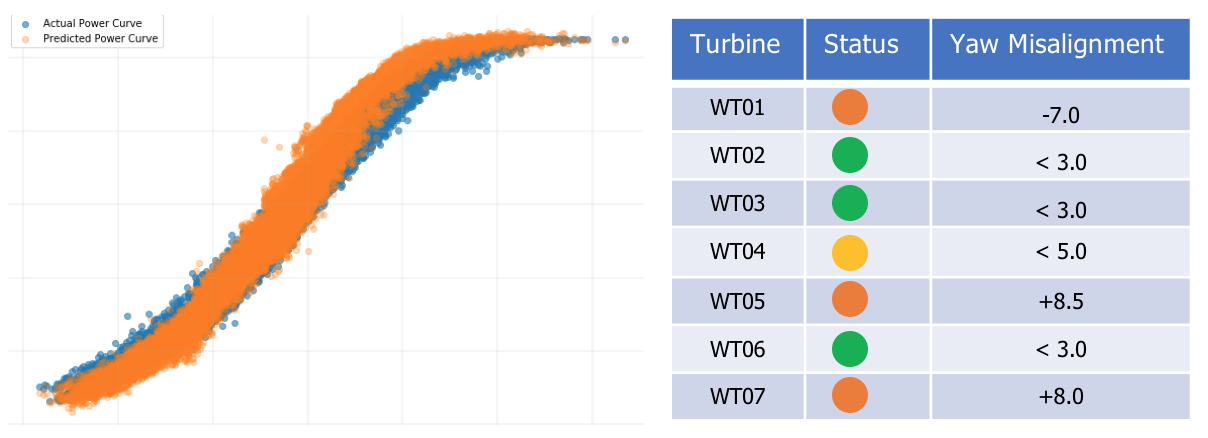
Energy.ML continuously monitors turbine production and compares the actual production to the expected production predicted by a machine learning model of historical turbine performance. Any deviations in turbine performance are automatically flagged and brought to the attention of the turbine operator, along with a diagnosis of the most likely factors responsible for the performance anomaly.
Generator Failure Prediction
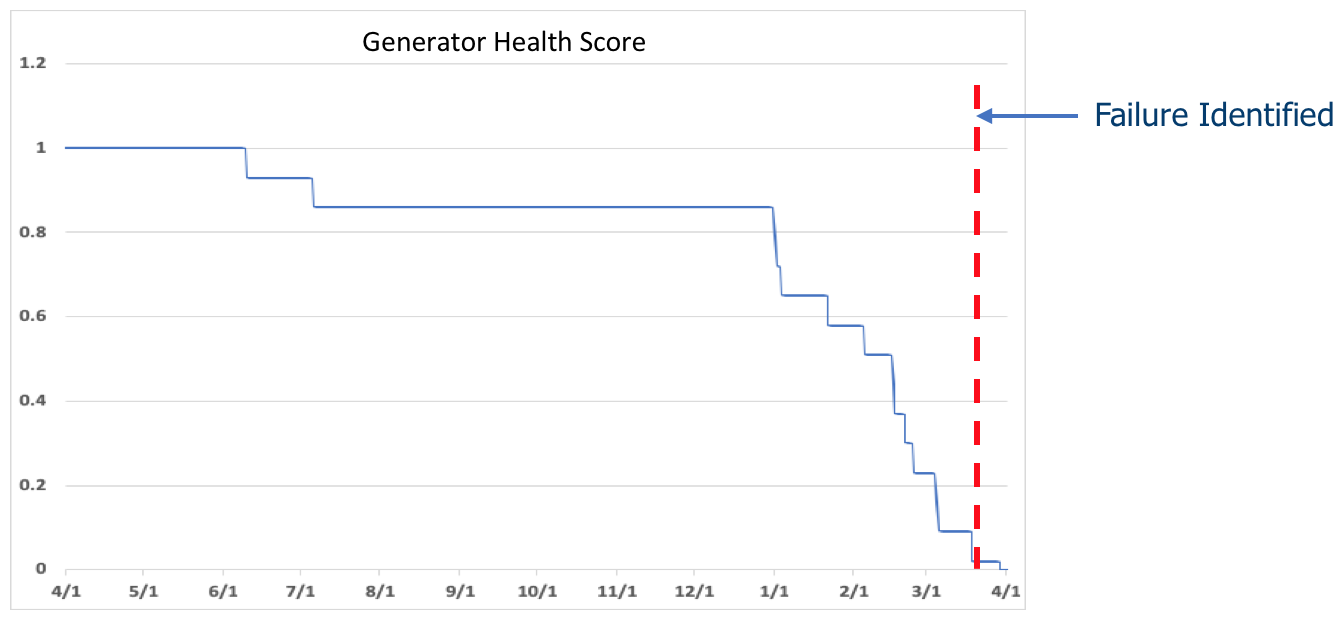
Many wind turbine generator failures result from failure of the generator stator insulation system. The predictive models in Energy.ML can predict generator stator insulation failures well in advance. In the example here, the Energy.ML generated health score of the generator dropped significantly three months in advance of the failure. The advance notification of the pending failure allowed our customer to get a new generator on order and make plans for a replacement, significantly reducing the machine downtime from the failure.